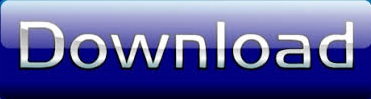
- #Mapping pandas data types to redshift data types for free#
- #Mapping pandas data types to redshift data types how to#
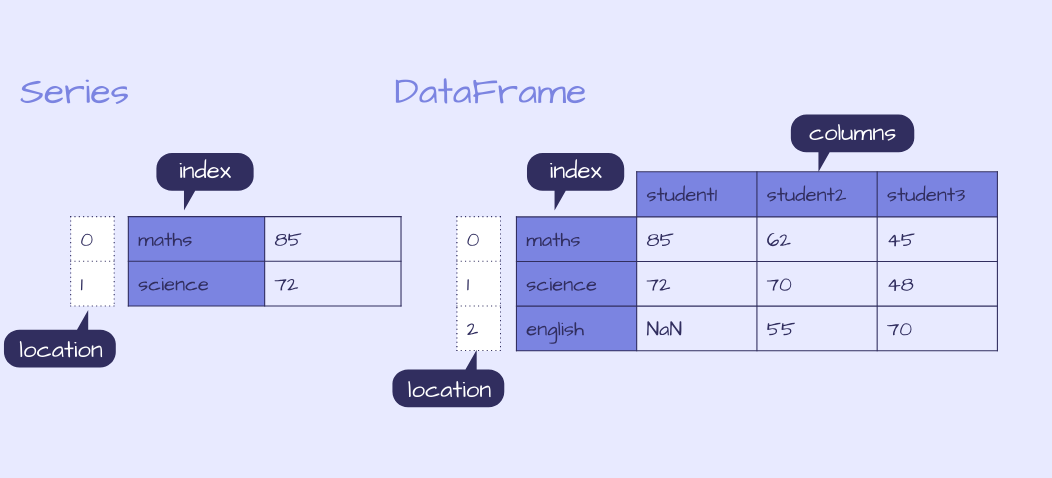
#Mapping pandas data types to redshift data types how to#
Use this for dealing with time across various time zonesīelow are some examples of how to use the above data types in your code.Įnter fullscreen mode Exit fullscreen mode Use this for dealing with time AND where your entire data warehouse is in the same timezone Use this for dealing with time spanning as small as a day, ie.
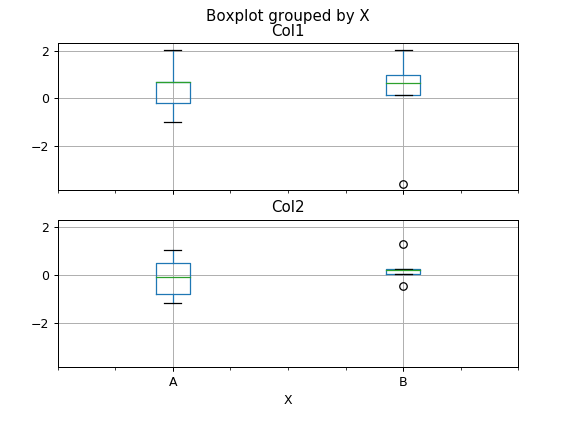
VARCHAR will always use up at least 6 bytes of data, even if you specify VARCHAR(2) So a in a VARCHAR(100) the word 'Christmas' will only use 9 of that 100, saving space. Unlike CHAR it will only use however much space has been entered.
#Mapping pandas data types to redshift data types for free#
VARCHAR allows for varying character length which is good for free text fields. VARCHAR, CHARACTER VARYING, NVARCHAR, TEXT CHAR will always use up at least 4 bytes of data, even if you specify CHAR(2) Use when your column is always going to be a fixed length. Which can cause issues with EXACT object matching. CHAR always takes up all of the space you specify, so if you specify char(100) but only put 'Hi' into the column, the remain 98 characters of space will be filled with spaces. You can specify that the default value is true or false, if you don't specify a default value then the default value will be nullĬHAR is a fixed length text string, ignore references to NCHAR and BPCHAR those are old functionality merged all into one.
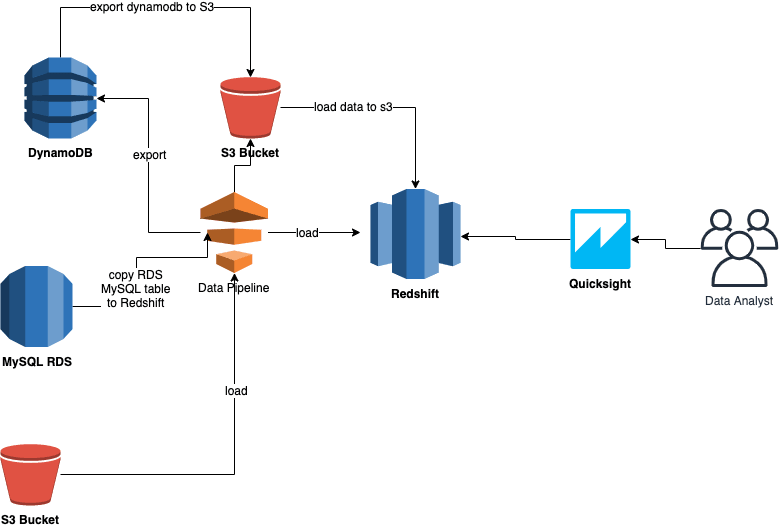

It can also get represented as a checkbox. For example decimal(10,2) means ten numbers max with two decimal places, this equates to 8 digits on the left of the decimal, and 2 on the right.įor storing smaller, rounded down, floating point numbersįor storing larger, non rounded, floating point numbersīoolean is a single byte flag which is either 1 or 0, true or false. When you classify a column as decimal you must declare both the TOTAL length and then how many decimals. This is for WHOLE numbers that only take up 2 bytes of data, range: -32768 to +32767Īlso for whole numbers that only take up 4 bytes of date, range: -2147483648 to +2147483647Īlso for whole numbers that only take up 8 bytes of date, range: -9223372036854775808 to 9223372036854775807įor numbers with decimal points, up to 38 digits total.
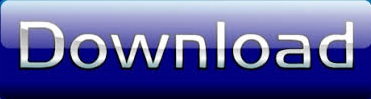